
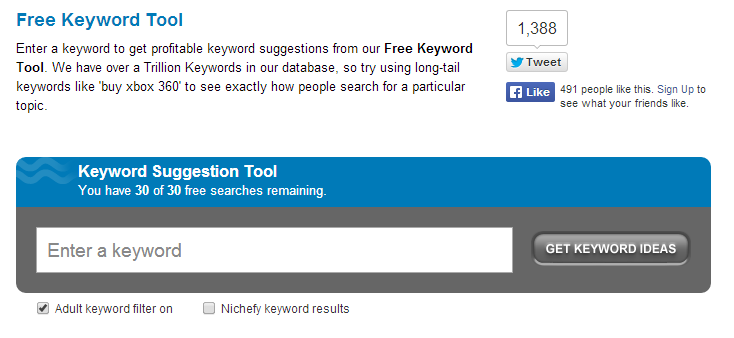

Spark SQL can turn on and off AQE by as an umbrella configuration. Adaptive Query ExecutionĪdaptive Query Execution (AQE) is an optimization technique in Spark SQL that makes use of the runtime statistics to choose the most efficient query execution plan, which is enabled by default since Apache Spark 3.2.0. SELECT /*+ REPARTITION_BY_RANGE(3, c) */ * FROM tįor more details please refer to the documentation of Partitioning Hints. SELECT /*+ REPARTITION_BY_RANGE(c) */ * FROM t Note that there is no guarantee that Spark will choose the join strategy specified in the hint sinceĪ specific strategy may not support all join types. Pick the build side based on the join type and the sizes of the relations. When both sides are specified with the BROADCAST hint or the SHUFFLE_HASH hint, Spark will When different join strategy hints are specified on both sides of a join, Spark prioritizes theīROADCAST hint over the MERGE hint over the SHUFFLE_HASH hint over the SHUFFLE_REPLICATE_NL With ‘t1’ as the build side will be prioritized by Spark even if the size of table ‘t1’ suggestedīy the statistics is above the configuration. For example, when the BROADCAST hint is used on table ‘t1’, broadcast join (eitherīroadcast hash join or broadcast nested loop join depending on whether there is any equi-join key) Instruct Spark to use the hinted strategy on each specified relation when joining them with another The join strategy hints, namely BROADCAST, MERGE, SHUFFLE_HASH and SHUFFLE_REPLICATE_NL, This configuration is only effective when using file-based data sources such as Parquet, ORC Paths is larger than this value, it will be throttled down to use this value. parallelismĬonfigures the maximum listing parallelism for job input paths. Using file-based data sources such as Parquet, ORC and JSON. This configuration is only effective when Otherwise, it will fallback to sequential listing. Input paths is larger than this threshold, Spark will list the files by using Spark distributed job. thresholdĬonfigures the threshold to enable parallel listing for job input paths. Statistics are only supported for Hive Metastore tables where the commandĪNALYZE TABLE COMPUTE STATISTICS noscan has been run.Ĭonfigures the number of partitions to use when shuffling data for joins or aggregations. By setting this value to -1 broadcasting can be disabled. Timeout in seconds for the broadcast wait time in broadcast joinsĬonfigures the maximum size in bytes for a table that will be broadcast to all worker nodes when This configuration is effective only when using file-based The suggested (not guaranteed) minimum number of split file partitions. This configuration is effective only when using file-based sources such as Parquet, Then the partitions with small files will be faster than partitions with bigger files (which is This is used when putting multiple files into a partition. The estimated cost to open a file, measured by the number of bytes could be scanned in the same This configuration is effective only when using file-based sources such as Parquet, JSON and ORC.
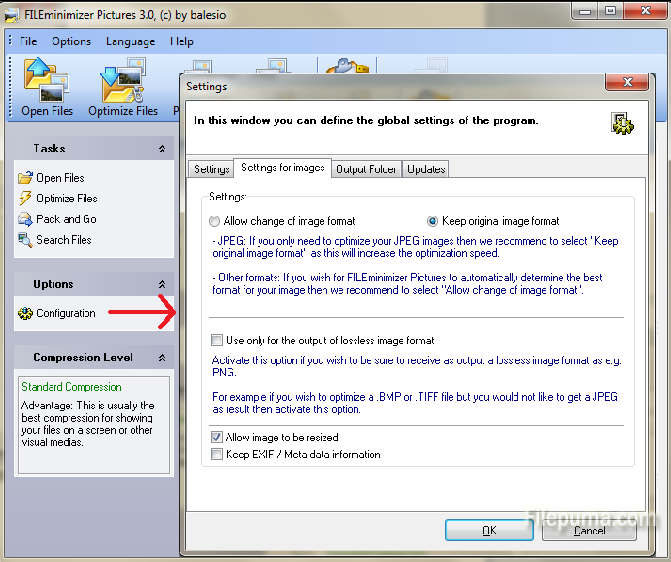
The maximum number of bytes to pack into a single partition when reading files. That these options will be deprecated in future release as more optimizations are performed automatically. The following options can also be used to tune the performance of query execution. Larger batch sizes can improve memory utilizationĪnd compression, but risk OOMs when caching data. 圜olumnarStorage.batchSizeĬontrols the size of batches for columnar caching. When set to true Spark SQL will automatically select a compression codec for each column based

You can call ("tableName") or dataFrame.unpersist() to remove the table from memory.Ĭonfiguration of in-memory caching can be done using the setConf method on SparkSession or by running Then Spark SQL will scan only required columns and will automatically tune compression to minimize Spark SQL can cache tables using an in-memory columnar format by calling ("tableName") or dataFrame.cache(). Converting sort-merge join to shuffled hash joinįor some workloads, it is possible to improve performance by either caching data in memory, or by.Converting sort-merge join to broadcast join.
